Details
Presenter(s)
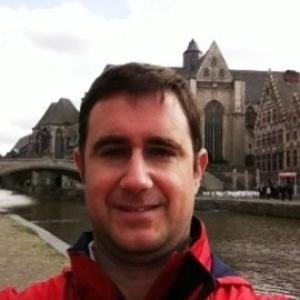
Display Name
Jorge Fernández-Berni
- Affiliation
-
AffiliationInstituto de Microelectrónica de Sevilla (CSIC-US)
- Country
Abstract
Convolutional Neural Networks (ConvNets) are directed acyclic graphs with node transitions determined by a set of configuration parameters. In this paper, we describe a dynamically configurable hardware architecture that enables data allocation strategy adjustment according to ConvNets layer characteristics. The proposed flexible scheduling solution allows the accelerator design to be portable across various scenarios of computation and memory resources availability. The system can autonomously adapt its behavior with no need of platform reconfiguration nor user supervision. Experimental results are presented and compared with state-of-the-art accelerators.