Details
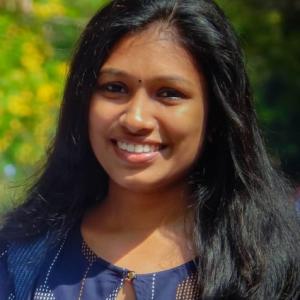
- Affiliation
-
AffiliationDigital University Kerala
- Country
Pruning is a process of removing unwanted neurons from neural network computations. In Neural Networks, pruning creates sparse information processing, which can improve the overall generalisation and energy efficiency of the network. By excluding redundant weight values which are not contributing significantly to the system performance, hardware complexity can be reduced while maintaining the recognition accuracy. This paper evaluates the effectiveness of unstructured pruning on memristive crossbar based neural nodes in Artificial Neural Network (ANN) and Binary Weighted Neural Network (BWNN) architectures. The impact of pruning is analysed in terms of inference accuracy, energy consumption and area efficiency. The robustness of the pruned system is validated under the influence of conductance variability, bit errors, and multiply and accumulate errors.