Details
Presenter(s)
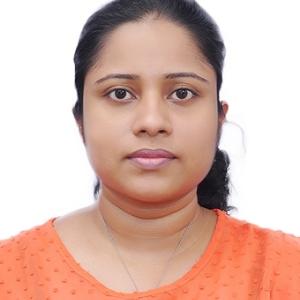
Display Name
Udari De Alwis
- Affiliation
-
AffiliationNational University of Singapore
- Country
-
CountrySingapore
Abstract
The diffusion of vision sensor nodes in a wide range of applications has given rise to higher computational demand at the edge of IoT. In vision applications, deep neural networks are well known to be a prime choice, in view of their performance and flexibility. However, such properties come at the cost of high computational requirements at inference time. In this paper, a computationally efficient inference technique is introduced to perform the task of object detection. The proposed method leverages the temporal correlation among frames, uniquely requires minor memory overhead for intermediate feature map storage, and does not require retraining.