Details
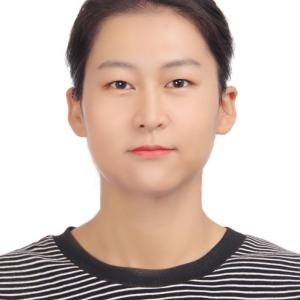
- Affiliation
-
AffiliationPeter Grünberg Institut 10, Forschungszentrum Jülich GmbH
- Country
-
CountryGermany
Cellular Nonlinear Networks (CNN) as a powerful paradigm is highly suitable for signal processing of multiple tasks, since they can execute cascaded processing operations in a one-layer array via real-time template updating. Their VLSI implementation by using the conventional CMOS-based integration technology, however, remains a big challenge. The memristive CNN (M-CNN) offers several merits over conventional CNN, such as compactness, nonvolatility, versatility. This paper presents a direct comparison of computing performance between the M-CNN and the conventional CNN for the implementation of a LOGAND operation template using circuit simulation. Our findings show that the M-CNN implementation offers faster convergence compared to the CNN implementation. In addition, the result is stored in a non-volatile manner in the M-CNN whereas the CNN only offers a volatile storage.