Details
Presenter(s)
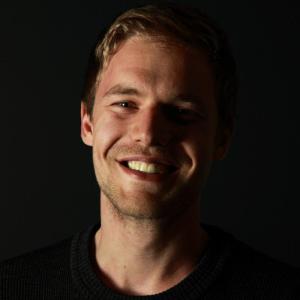
Display Name
Nik Dennler
- Affiliation
-
AffiliationUniversity of Hertfordshire (UK) and Western Sydney University (AUS)
- Country
Abstract
Information from vibration patterns in running industrial systems is commonly used for predictive maintenance. This information could also be exploited for smaller-scale robotic systems. Hardware spiking neural networks can provide a valuable technology for such use cases. Here we present a spike-based pipeline for detecting anomalies from vibration data, using primitives that are compatible with analog-digital neuromorphic hardware. This pipeline makes use of a cochlea model, feedback adaptation, and balanced excitatory-inhibitory networks. We show that the model achieves state-of-the-art performance against two standard data sets, and validate it on a neuromorphic processor.