Details
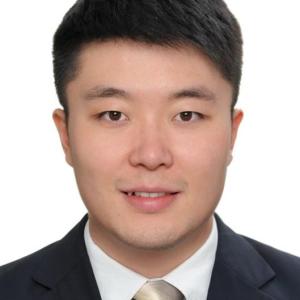
- Affiliation
-
AffiliationZhejiang University
- Country
-
CountryChina
AI inference based on novel compute-in-memory devices have shown clear advantages in terms of power, speed and storage density, making it a promising candidate for IoT and edge computing applications. In this work, we demonstrate a fully integrated system-on-chip (SoC) design with embedded Flash memories as the neural network accelerator. A series of techniques from device, design and system perspectives are combined to enable efficient AI inference for resource-constrained voice recognition. 7-bit/cell storage capability and self-adaptive write of novel Flash memories are leveraged to achieve state-of-the-art overall performance. Also, model deployment techniques based on transfer learning concepts are explored to significantly improve the accuracy loss during weight data deployment. Integrated in a compact form factor, the whole voice-recognition system can achieve >10 TOPS/W efficiency and ~95% accuracy for real-time keyword spotting application.