Details
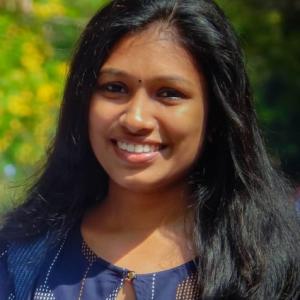
- Affiliation
-
AffiliationDigital University Kerala
- Country
The increasing demand for a high density of transistors in the chip with high yield often requires the manufacturing process to be mature. Defects are common when a new process technology is introduced. Manual testing for each wafer during a separate fabrication stage is a time-consuming process. To improve the production quality, the wafer map obtained after the electrical probe test should be categorized into corresponding defect classes. In this paper, we propose an automated wafer defect classification system using a Convolutional neural network (CNN)-memristor crossbar structure. The weights extracted from a pretrained neural network model are deployed into a crossbar structure. The output probability values from the softmax layer determine the class to which the wafer input belongs. The performance of CNN architecture is compared with other deep learning models. The area and power requirements of the proposed hardware architecture are also presented.