Details
Presenter(s)
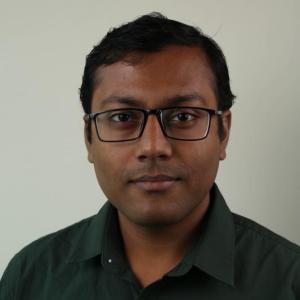
Display Name
Saion Kumar Roy
- Affiliation
-
AffiliationUniversity of Illinois at Urbana Champaign
- Country
Abstract
This paper determines the fundamental limits on the compute SNR of MRAM-, ReRAM-, and FeFET-based crossbars by employing statistical signal and noise models. The maximum compute SNR is shown to occur at an optimum value of sensing resistance. SNR can be further improved by choosing devices with higher resistive contrast, but only until the range 12-15. Beyond this, mismatch in the input digital-to-analog converters and bitcell variations begin to dominate the compute SNR. Finally, by mapping a ResNet-20 (CIFAR-10) network onto resistive crossbars, it is shown that the array-level compute SNR maximizing circuit parameters also maximizes the network-level accuracy.