Details
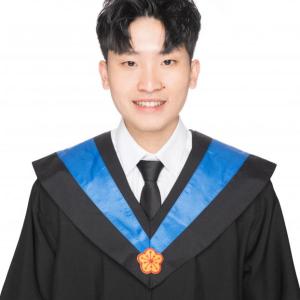
- Affiliation
-
AffiliationNational Taiwan University
- Country
Federated learning (FL) is a privacy-preserving learning framework. However, most FL works focus on deep neural networks (DNNs), whose intensive computation hinders FL from practical realization on resource-limited edge devices. In this paper, we exploit the high energy efficiency properties of hyperdimensional computing (HDC) to propose a federated learning HDC (FL-HDC). In FL-HDC, we bipolarize model parameters to significantly reduce communication costs, which is a primary concern in FL. Moreover, we propose a retraining mechanism with adaptive learning rates to compensate for the accuracy degradation caused by bipolarization. Compared with the previous work that transmits complete model parameters to the cloud, FL-HDC greatly reduces 23x and 9x communication costs with comparable accuracy in ISOLET and MNIST, respectively.