Details
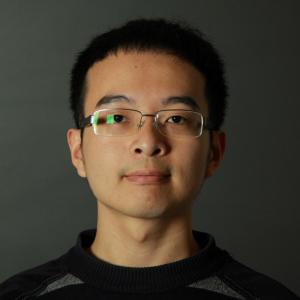
- Affiliation
-
AffiliationUniversity of Zürich and ETH Zürich
- Country
This paper proposes a fully-connected network training architecture called EILE targeting incremental learning on edge. By using a novel reconfigurable processing element (PE) architecture, EILE avoids explicit transposition of weight matrices required for backpropagation to preserve the same efficient memory access pattern for both the forward (FP) and backward propagation (BP) phases. Experimental results on a Zynq XC7Z100 FPGA with 64 PEs show that EILE achieves 19.2\,GOp/s peak throughput and maintains nearly 100\% PE utilization efficiency for both FP and BP with batch sizes from 1 to 32. EILE's small on-chip memory footprint and scalability to match any available off-chip memory bandwidth makes it an attractive ASIC architecture for energy-constrained training.