Details
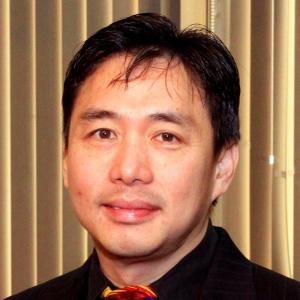
- Affiliation
-
AffiliationIBM T. J. Watson Research Center
- Country
We present an application of machine learning to automated robust optimization of electronic circuit design that combines artificial neural networks and global optimization. A neural network regressor is constructed to predict circuit operation metrics such as power, offset, delay, phase margin based on input parameters such as device size, temperature, supply voltage and current, and process corner. This regressor is then used to build an objective function for global optimization to find the optimal set of controllable parameters that optimize the objective function subject to input constraints such as device size range and output constraints such as power consumption or delay. Experimental results from tuning state-of-the-art high performance PLL circuits show that this framework is promising and can in much less time find optimized circuits that outperform circuit designs that were manually tuned by a skilled circuit designer.