Details
Presenter(s)
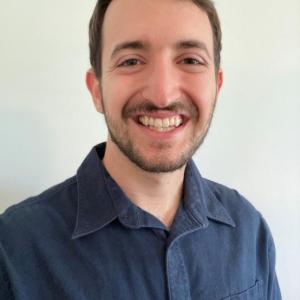
Display Name
Nathan Eli Miller
- Affiliation
-
AffiliationGeorgia Institute of Technology
- Country
-
CountryUnited States
Abstract
We analyze the impact of drain current variation in 28 nm high-K metal-gate Ferroelectric FETs on FeFET-based processing-in-memory deep neural network accelerators. Non-Normal variation in drain current is observed from repeated read operation on FeFETs with different channel dimensions at various read frequencies. Device-circuit co-analysis using the measured current distribution shows a 1 to 3 percent accuracy degradation of an FeFET-based PIM platform classifying the Fashion-MNIST dataset with the LeNET-5 DNN. This accuracy drop can be fully recovered with variation-aware training, showing that individual FeFET current variation over many read cycles is not prohibitive to the design of DNN accelerators.