Details
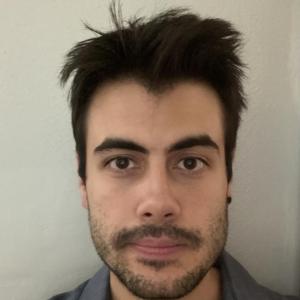
- Affiliation
-
AffiliationUniversity of Wisconsin - Madison
- Country
Freezing of gait (FoG), which implies a brief absence or reduction of ability to walk, is one of the most common symptoms of Parkinson\'s Disease (PD). Predicting FoG episodes in time can prevent their onset by providing specific cues to the patients. This paper presents a deep learning approach to predict FoG episodes using a Long Short-Term Memory network (LSTM). It also identifies key issues and concepts which have not been dwelled upon before in the existing literature, paving the way to a more systematic methodology for future work. We evaluate our approach using a publicly available dataset that includes accelerometer readings from 10 PD patients. We achieve up to 89% prediction accuracy with an average prediction time of 1.42 s using a subject-independent model.