Details
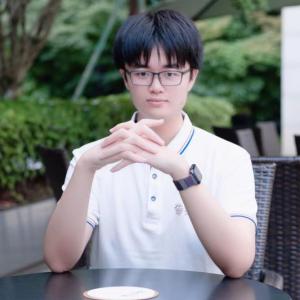
- Affiliation
-
AffiliationFudan University, Hong Kong University of Science and Technology
- Country
-
CountryChina
EMG based hand gesture recognition on convolutional neural networks (CNNs) has been widely learned, which gains high accuracy. However, CNN based systems are computationally complex and power consuming, thus hard to be deployed at edge. Biologically inspired, a new neuromorphic learning and computing approach for EMG based hand gesture recognition tasks is proposed in this work. This approach designs an activate and inhibit joint processing spiking neural network (AIPS-SNN) which reaches an accuracy of 85.6% on Nina Pro dataset. Furthermore, the AIPS-SNN is deployed on the proposed memristor based computation in-memory (CIM) system, the power efficiency and area efficiency of which reach 10.146 TOPS/W and 35.399 GOPS/mm2, respectively. The experimental results indicate that the proposed neuromorphic CIM engine is promising for edge deployment.