Details
Poster
Presenter(s)
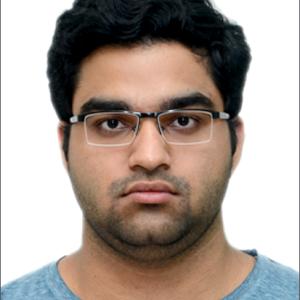
Display Name
Shikhar Tuli
- Affiliation
-
AffiliationIndian Institute of Technology Delhi
- Country
Abstract
In this work we use Machine Learning to enhance the capabilities of present RRAM controllers. The proposed Adaptive RRAM Variability-Aware Controller, AVAC, updates Wait Buffer and batch sizes after every interval using novel on-the-fly learning polynomial models with gradient ascent. AVAC allows Edge devices to adapt to different applications and their stages, to increase computation performance and reduce energy consumption. We demonstrate that the proposed model can provide up to 3% increase in performance and 89% decrease in energy, compared to static controllers, for real-life healthcare applications on a Raspberry-Pi based Edge deployment.