Details
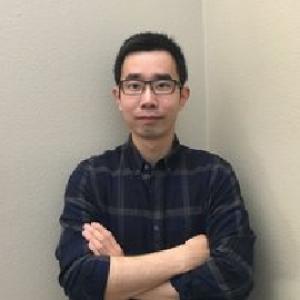
- Affiliation
-
AffiliationUniversity of California, Irvine
- Country
-
CountryUnited States
Training Deep Recurrent Neural Networks (Deep-RNNs) using Back Propagation Through Time (BPTT) has shown tremendous success in improving benchmark performance and solving real-world problems. However, the non-locality of loss functions for deep networks and the requirement of parallel computing hardware to propel the learning make it challenging to map gradient-based RNNs onto neuromorphic devices. This study improves the stability of a neuromorphic-friendly RNN called full-FORCE by dynamically coupling targets with the network and introducing a multi-layer architecture. The proposed network outperforms the original version on both pattern generation and classification in biomedical signals such as electroencephalogram (EEG) for brain-interfacing devices.