Details
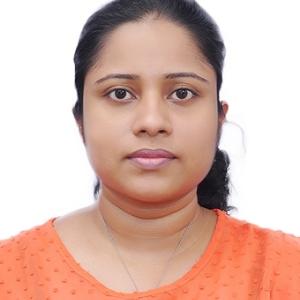
- Affiliation
-
AffiliationNational University of Singapore
- Country
-
CountrySingapore
Making sense of human actions in video sequences has become an essential task in video surveillance applications. In such applications, 3D CNNs are a prime choice thanks to their excellent performance. However, the performance advantage offered by these networks comes at a significant computational and memory cost. In this paper, a novel 3D CNN accelerator architecture which leverages on temporal similarity to reduce computations is introduced. The architecture is analyzed and validated with video benchmarks for human action recognition. The proposed Temporal Similarity Removal (TSR ) accelerator reduces computation in the convolutional layers of a 3D CNN by skipping feature map similarities introduced by Temporal Similarity Tunnels (TST) among adjacent frames. The proposed architecture achieves 2x better area efficiency and 55%-3.5x (45%) better energy efficiency over prior art, based on the C3D network (3D MobileNet network) and the UCF101 dataset.