Details
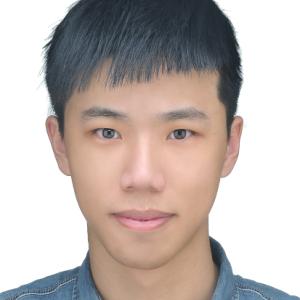
- Affiliation
-
AffiliationNational Changhua University of Education
- Country
Acceleration and Reliability are two critical issues of artificial intelligent circuits and systems. In this paper we propose a novel structure of deep neural network in testing stage that converts the trained weights to optimized ternary-coded binary. Within each shallow layer a constant-shift sub-layer with almost-free cost is inserted to transfer all multipliers to a carry save adder/subtractor. Then the activation functions are simplified to piecewise lines with nice slopes for calculation by an adder only. Since input-side layers tend to have self-healing ability, only output-side layers are equipped by AN codes that can be encoded and decoded almost without extra cost in our structure. From experiments and evaluations, our structure can have a higher resolution with error-correcting capability and a performance similar to the BNN.