Details
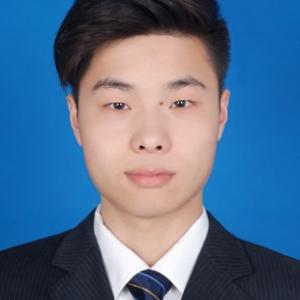
- Affiliation
-
AffiliationSoutheast University
- Country
-
CountryChina
Spiking-Neural-Networks (SNN) have natural advantages in high-speed signal processing and big data operation. However, due to the complex implementation of synaptic arrays, SNN based accelerators may face low area utilization and high energy consumption. Computing-In-Memory (CIM) shows great potential in performing intensive and high energy efficient computations. In this work, we proposed a 10T-SRAM based Spiking-Neural-Network-In-Memory architecture (SNNIM) with 28nm CMOS technology node. A compact 10T-SRAM bit-cell was developed to realize signed 5bit synapses arrays and configurable bias arrays (SYBIA). The soma array based standard 8T-SRAM (SMTA) stores the soma membrane voltage and the threshold value. A capacitance computation scheme (CCA) between them was proposed to support various SNN operations. The proposed SNNIM achieved energy efficiency of 25.18 TSyOPS/W. And the proposed SNNIM achieved 1.79+× better array efficiency compared with previous works.