Details
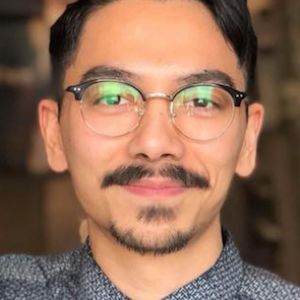
- Affiliation
-
AffiliationUniversité Laval
- Country
This work proposes a framework to train and deploy neural network-based gesture recognition algorithms in wearable devices. The approach is demonstrated for a high-density electromyography (HD-EMG) gesture recognition system where a siamese convolution neural network (SCNN) learns to associate and dissociate muscle activity patterns from same and distinct gesture classes. This optimizes learning in low-data environments such as gesture recognition and myoelectric control where training data has to be provided by the end user. Then using a cosine similarity few-shot classifier and inter-session-intra-user transfer of the SCNN’s learning, the proposed model is intended to achieve state-of-the-art results in a framework that is realistically applicable in wearable devices. For an experimented myoelectric interface user, the proposed model achieved 89.24 % accuracy in 6-class gesture recognition with inter-session-intra-user transfer learning of the SCNN. An accuracy of 84.05 % was achieved with 8-shot learning on the cosine similarity classifier.