Details
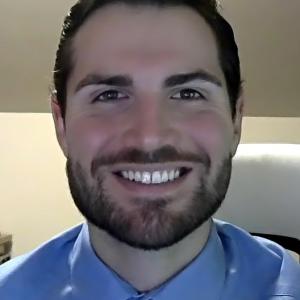
- Affiliation
-
AffiliationUniversity of Windsor
- Country
The concept of input sparsity in Spiking Neural Networks for pattern recognition is introduced and explored with the goals of reductions in network inference time, network size, and in hardware implementations reductions in resource requirements. A method is proposed by which selective input sparsity can be inferred from the training set to reduce the size of the network before training and decrease the network inference time while simultaneously increasing the network\'s inference accuracy. For a basic fully connected spiking neural network trained to solve the MNIST handwritten digits, selective input sparsity is applied and the network size is reduced by 76.15% with a 3.87% improvement in the classification accuracy and a 75.88% decrease in the network\'s inference time.