Details
Presenter(s)
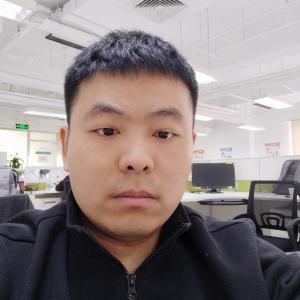
Display Name
Xin Wang
- Affiliation
-
AffiliationInstitute of Information Engineering, University of Chinese Academy of Sciences, CAS
- Country
Abstract
A major challenge in knowledge base question answering tasks is to obtain answers in a large search space, while the dataset contains limited training data. To address this challenge, we propose a novel method, adopting few-shot learning to enable the KBQA model to find golden answers more precisely with limited samples. To achieve this, we design a question embedding model to retrieve similar historical questions to the target question and utilize prior features from the retrieved questions to make the KBQA model search answers more concisely. Experimental results on the benchmark datasets have demonstrated the effectiveness of our approach.