Details
Presenter(s)
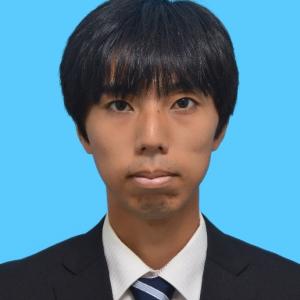
Display Name
Kengo Nakata
- Affiliation
-
AffiliationKioxia Corporation
- Country
-
CountryJapan
Abstract
Quantization is an effective technique to reduce memory and computational costs for inference of convolutional neural networks. However, it has not been clarified which model achieves better recognition accuracy with lower memory and computational costs: large models quantized with extremely low bit precision or small models quantized with moderately low bit precision. To answer this question, we define a metric that combines the number of parameters and computations with bit widths of quantized parameters. Using this metric, we demonstrate that small and moderately quantized models achieve better accuracy with low memory and computational costs than large and extremely quantized models.