Details
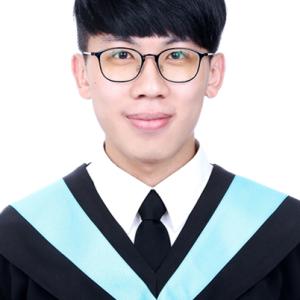
- Affiliation
-
AffiliationNational Yang Ming Chiao Tung University
- Country
Computing-in-memory (CIM) has attracted significant attentions in recent years due to its massive parallelism and low power consumption. However, current CIM designs suffer from large area overhead of small CIM macros and bad programmablity for model execution. This paper proposes a programmable CIM processor with single large sized CIM macro instead of multiple smaller ones for power efficient computation and flexible instruction set to support various binary 1-D convolution Neural Network (CNN) models in a easy way. Furthermore, the proposed architecture adopts the pooling write back method to support fused or independent convolution/pooling operations to reduce 35.9\\% of latency, and the flexible ping-pong feature SRAM to fit different feature map size during layer-by-layer execution. The design fabricated in TSMC 28nm technology achieves 150.8 GOPS throughput and 885.86 TOPS/W power efficiency at 10 MHz when executing our binary keyword spotting model, which has higher power efficiency and flexibility than previous designs.