Details
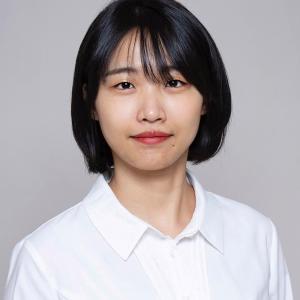
- Affiliation
-
AffiliationETH Zürich
- Country
Energy harvesting can enable wireless smart sensors to be self-sustainable by allowing them to gather energy from the environment. However, since the energy availability changes dynamically depending on the environment, it is difficult to find an optimal energy management strategy at design time. One existing approach to reflecting dynamic energy availability is energy-aware adaptive sampling, which changes the sampling rate of a sensor according to the energy state. This work proposes deep reinforcement learning-based predictive adaptive sampling for a wireless sensor node. The proposed approach applies deep reinforcement learning to find an effective adaptive sampling strategy based on the harvesting power and energy level. In addition, the proposed approach enables predictive adaptive sampling by designing adaptive sampling models that consider the trend of energy state. The evaluation results show that the predictive models can successfully manage the energy budget reflecting dynamic energy availability, maintaining a stable energy state for a up to 11.5% longer time.