Video Not Available
Details
Presenter(s)
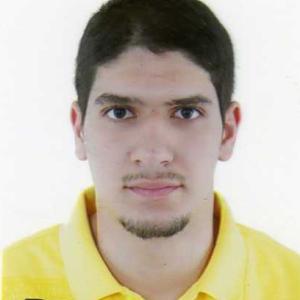
Display Name
Khalil Chikhaoui
- Affiliation
-
AffiliationKing Fahd University of Petroleum and Minerals
- Country
Abstract
Autonomous navigation of unmanned aerial vehicles (UAVs) in urban environments is a difficult task due to the complexity of the surroundings and the battery limitations of the flying unit. In this paper, we present an autonomous framework for UAV navigation using deep reinforcement learning. We deploy a proximal policy optimization (PPO) algorithm for path planning with collision avoidance. The energy limitations of the UAV is taken into account to prevent crashes due to low battery situations. We develop a virtual environment that simulates a random three dimensional space with obstacles, and we use it to train the model and evaluate its performance. It is shown that 90% of the trips have been successfully accomplished.