Details
Presenter(s)
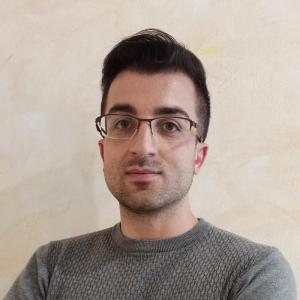
Display Name
Carmine Paolino
- Affiliation
-
AffiliationPolitecnico di Torino
- Country
Abstract
In this work, we describe a methodology that, starting from measurements performed on a set of real PCM devices, enables the training of a neural network. The core of the procedure is the creation of a computational model, sufficiently general to include the effect of unwanted nonidealities. Results show that, depending on the task at hand, a different level of accuracy is required in the PCM model applied at train-time to match the performance of a traditional, reference network. Moreover, the trained networks are robust to the perturbation of the weights.