Details
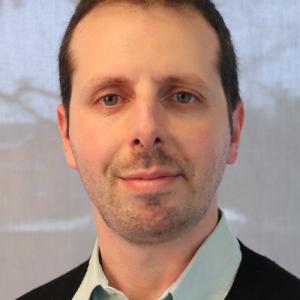
- Affiliation
-
AffiliationGeorge Mason University
- Country
Multiple user authentication techniques including cryptographic and biometric approaches are introduced for authentication of the users on Internet-of-Things (IoT) devices. Despite effective,these techniques incur large computational and communication overheads. In contrast, we propose a gait-based authentication,suitable for IoT devices in this work. Across multiple gait signals,we consider walking gait in this work, as it is unique to every individual and can be measured in an unobtrusive manner by utilizing the inherent sensors, which are inherently embedded in the IoT devices as well as smartphones. Given the limited resources available on IoT devices, we propose a lightweight authentication method that allows for early exit from the Neural Network (NN) in order to optimize the computational costs. A Deep Q-Learning Network (DQN) reinforcement learning method is further introduced to determine the exit dynamically during the authentication. The proposed method has been evaluation on the whuGAIT dataset.