Details
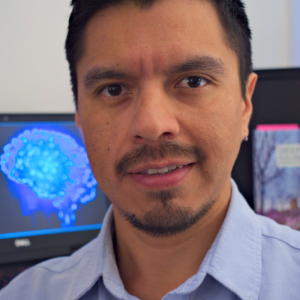
- Affiliation
-
AffiliationUniversity of Saskatchewan
- Country
The inclusion of technology in agriculture is highly relevant given the increasing global demand for food and our growing population. This paper focuses on an application for the analysis of multispectral images of wheat fields, and how they could be used to predict yield in a way that is resource-efficient and time convenient. Thus, our main goal is to optimize a deep learning model already proposed in the literature, through feature selection, pruning, and quantization, to be efficient enough that it could be deployed on a computer with limited resources. The main results of this work show that the size of the model was reduced by almost 94%, its inference time was almost 73% faster compared to the original model, while reducing its performance by 19% (still better than what was found in literature). This could be an important step towards the deployment of edge intelligence for plant phenotyping.