Details
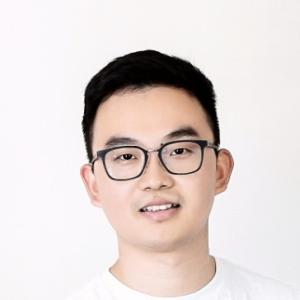
- Affiliation
-
AffiliationBytedance Inc.
- Country
The optimized bit allocation among frames has been intensively explored and improved the compression performance significantly in conventional video coding. However, the optimized bit allocation is still in its infant stage for learning-based video coding. Most existing learning-based video compression methods either use uniform bit allocation or empirically determined bit allocation weights among frames. In this paper, we develop an optimized bit allocation scheme for learning-based end-to-end video compression. In particular, we realize a hierarchical quality-control mechanism based on the importance of different frames under random-access scenarios. Considering the varying importance of frames on different temporal layers, we propose an efficient yet simple scheme, in which a set of optimized bit allocation weights are introduced to the rate-distortion (R-D) loss function. Experimental results demonstrate the effectiveness of the proposed scheme. In addition, the proposed scheme can be easily applied to most existing learning-based video compression frameworks under random-access scenarios.