Details
Presenter(s)
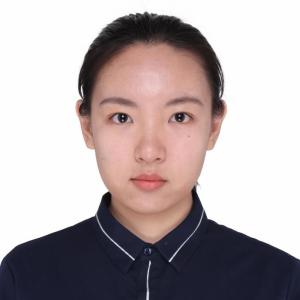
Display Name
Anni Lu
- Affiliation
-
AffiliationGeorgia Institute of Technology
- Country
-
CountryUnited States
Abstract
Compute-in-memory (CIM) is an attractive solution to deep neural network (DNN) hardware accelerators. DNN+NeuroSim is an integrated benchmark framework for fast early-stage design space exploration of CIM accelerators supporting flexible and hierarchical design options from device-level to circuit-level and up to algorithm-level. In this paper, we validate and calibrate the prediction of NeuroSim against a 40nm RRAM-based CIM macro post-layout simulations for area, latency and energy. Some adjustment factors are introduced to account for wiring area in layout, gate switching activity and post-layout performance drop, etc. The prediction from NeuroSim is precise with chip-level error under 1% after the calibration.