Details
Presenter(s)
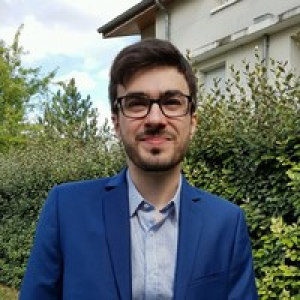
Display Name
Julien Posso
- Affiliation
-
AffiliationPolytechnique Montréal
- Country
Abstract
Spacecraft pose estimation is an essential computer vision application that can improve the autonomy of in-orbit operations. An ESA/Stanford competition brought out solutions that seem hardly compatible with the constraints imposed to spacecraft onboard computers. Among the best in the competition, URSONet stands out for its generalization capabilities, but at the cost of a tremendous number of parameters and high computational complexity. In this paper, we propose Mobile-URSONet, a spacecraft pose estimation convolutional neural network that has as little as 178 times fewer parameters while degrading accuracy by no more than 3 times compared to URSONet.