Details
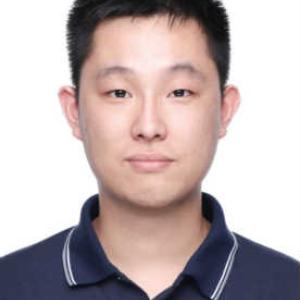
- Affiliation
-
AffiliationImperial College London
- Country
Blood glucose (BG) prediction is crucial to the successful management of type 1 diabetes (T1D) by allowing for proactive medical interventions and treatment. We present an IoT-enabled wearable device for real-time BG prediction based on continuous glucose monitoring (CGM) and a novel attention-based recurrent neural network (RNN). The complete hardware contains a system on a chip (SoC) that enables BLE connectivity and executes the embedded RNN with edge inference. This device can provide 24-hour predictive glucose alerts, i.e., hypoglycemia, to improve BG control and prevent or mitigate potential complications. Meanwhile, it can be connected to desktop computers and smartphones for the visualization of BG trajectories, data storage, and model update.