Details
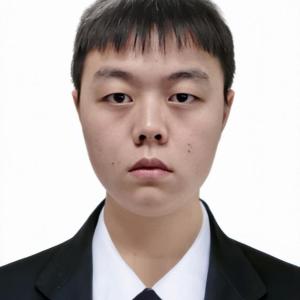
- Affiliation
-
AffiliationSouthwest University of Science and Technology
- Country
-
CountryChina
With the rapid development of deep learning, convolutional neural networks(CNNs) have achieved great success. But these high-capability CNNs often with a huge burden of computation and memory, which hinders these CNNs from applying to practical application. To solve this problem, in this paper, we proposed a method to train a compact model with high-capacity. The student network with fewer parameters and calculations will learning from the knowledge of the teacher network with more parameters and calculations. To promote the ability of the student network, the more expressive knowledge is extracted from the middle-layer feature of neural networks by attention mechanism, and the knowledge transforms more effective from the teacher network to the student network by the positive-unlabeled(PU) classifier. We validate our method in extensive experiments, showing that it can train the student network to achieve significant performance superior to the teacher network.