Details
Presenter(s)
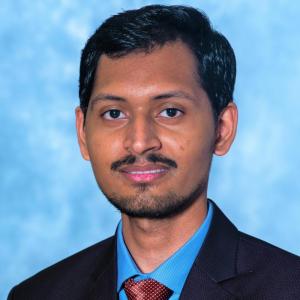
Display Name
Md Hasibul Amin
- Affiliation
-
AffiliationUniversity of South Carolina
- Country
Abstract
We investigate the effect of interconnect parasitic on the accuracy of deep neural networks (DNNs) deployed on fully-analog in-memory computing (IMC) architectures. Moreover, we propose a mechanism to alleviate the parasitic impacts by dividing large arrays into multiple partitions. The SPICE simulation results for a 400X120X84X10 DNN model deployed on a partitioned fully-analog IMC circuit show a 94.84% classification accuracy for the MNIST dataset, which is comparable to the ~97% accuracy realized by digital implementation on CPU. Results show that accuracy benefits are achieved at the cost of higher power consumption due to the extra circuitry required for handling partitioning.