Details
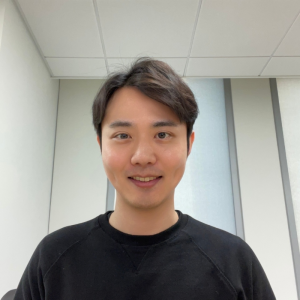
- Affiliation
-
AffiliationSeoul National University
- Country
Due to the fundamental limit to reducing power consumption of running deep learning models on von-Neumann architecture, research on neuromorphic computing systems based on low-power spiking neural networks using analog neurons is in the spotlight. In order to integrate a large number of neurons, neurons need to be designed to occupy a small area, but as technology scales down, analog neurons are difficult to scale, and they suffer from reduced voltage headroom/dynamic range and circuit nonlinearities. In light of this, this paper first models the nonlinear behavior of existing current-mirror-based voltage-domain neurons designed in a 28nm process, and show SNN inference accuracy can be severely degraded by the effect of neuron\'s nonlinearity. Then, to mitigate this problem, we propose a novel neuron, which processes incoming spikes in the time domain and greatly improves the linearity, thereby improving the inference accuracy compared to the existing voltage-domain neuron. Tested on the MNIST dataset, the inference error rate of the proposed neuron differs by less than 1% from that of the ideal neuron.