Details
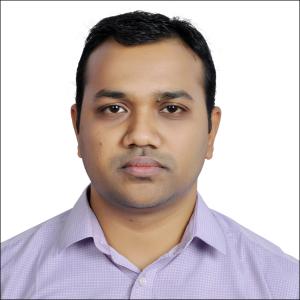
- Affiliation
-
AffiliationKhalifa University
- Country
The long-term deployment of data-driven AI technology using artificial neural networks (ANNs) should be scalable and re-deployable when new data becomes available. For such efficient adaptation, the learning should be cumulative so that new data are processed within the modified network without compromising the inference performance of past data. Such incremental accumulation of learning experience is known as progressive learning. In this paper, we address the open problem of tuning the hyper-parameters of neural networks during task progressive learning. A hyper-parameter optimization framework is proposed that selects the best value of hyper-parameters on a task-by-task basis. The neural network model adapts to each progressive learning task by adjusting the hyper-parameters under which the neural architecture is incrementally grown. Several hyper-parameter search mechanisms are explored and compared in support of progressive learning. The proposed methodology has been successfully demonstrated using a set of cyber-security datasets.