Details
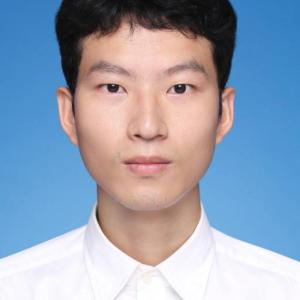
- Affiliation
-
AffiliationFudan University
- Country
Photometric stereo aims at recovering the surface and shape of an object from a set of observations under different light conditions. However, the shapes, materials, and reflectance properties of objects in these datasets are limited, leading to abridged performances in realistic environments. In this paper, we introduce a work-piece dataset for near-light photometric stereo under industrial application scenarios, which consists of observed images taken under at most 40 light conditions, and the ground truth surface normals. Based on this datasets, we propose the Hybrid Near-light Uncalibrated Photometric Stereo (HNUPS) for both unsupervised light calibration and surface normal estimation. Experimental results on the work-piece dataset demonstrate that HNUPS can obtain the least mean angular error when compared to recent deep learning methods, which has verified the effectiveness of the proposed HNUPS.