Details
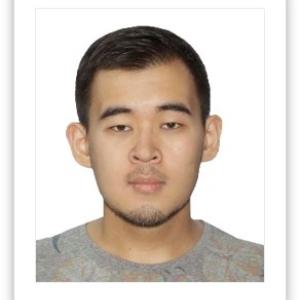
- Affiliation
-
AffiliationUniversity of Delaware
- Country
Spiking Neural Networks shows promising results as the architecture of choice for realizing neuromorphic circuits based on emerging nonvolatile memory devices. High classification performance of Convolutional Neural Networks (CNNs) in processing of unstructured visual data render Spiking Convolutional Neural Networks (SCNNs) as the preferred architecture for energy-efficient visual data processing on neuromorphic systemon- a-chip. Mapping of CNN operation to CMOS/RRAM arrays has recently gained attention but the prior proposed architectures/ circuits have been realized with incomplete functionality and peripheral circuit considerations. Max-Pooling is an essential operation in an SCNN layer, but it incurs significant area overhead when implemented directly in RRAM/CMOS. In this work, we propose a novel area-efficient SCNN circuit with temporal Max-Pooling and integrator sharing across the input features. Transistor-level simulations of the proposed SCNN realized on a crossbar array with peripheral circuits are presented using a hybrid 130nm CMOS technology with BEOL integrated HfOx RRAM devices