Details
Presenter(s)
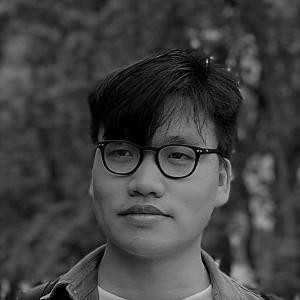
Display Name
Van Thien Nguyen
- Affiliation
-
AffiliationCEA-Leti
- Country
-
CountryFrance
Abstract
Adjusting the quantization according to the data or to the model loss seems mandatory in order to enable a high accuracy in the context of quantized neural networks. This work presents Histogram-Equalized Quantization (HEQ), a novel adaptive framework for linear and symmetric quantization. HEQ automatically adapts the quantization thresholds using a unique step size optimization. We empirically show that HEQ achieves state-of-the-art performances on CIFAR-10. Moreover, experiments on the STL-10 dataset show that HEQ enables a proper training of our proposed logic-gated (OR, MUX) residual networks with a higher accuracy at a lower hardware complexity than previous work.