Details
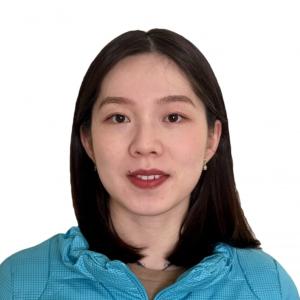
- Affiliation
-
AffiliationUniversity of Southern California
- Country
Being different from deep-learning-based (DL-based) image generation methods, a new image generative model built upon successive subspace learning principle is proposed and named GenHop (an acronym of Generative PixelHop) in this work. GenHop consists of three modules: 1) high-to-low dimension reduction, 2) seed image generation, and 3) low-to-high dimension expansion. In the first module, it builds a sequence of high-to-low dimensional subspaces through a sequence of whitening processes, each of which contains samples of joint-spatial-spectral representation. In the second module, it generates samples in the lowest dimensional subspace. In the third module, it finds a proper high-dimensional sample for a seed image by adding details back via locally linear embedding (LLE) and a sequence of coloring processes. Experiments show that GenHop can generate visually pleasant images whose FID scores are comparable or even better than those of DL-based generative models for MNIST, Fashion-MNIST and CelebA datasets.