Details
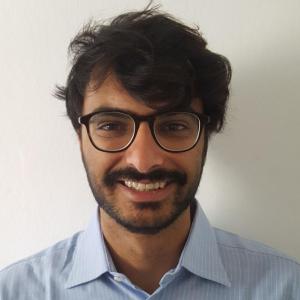
- Affiliation
-
AffiliationPolitecnico di Milano
- Country
Deep learning models have reached high accuracy in multiple classification tasks. However these models lack explainability. On the other hand, tree-based models are top performers in several applications, particularly when the training set is limited, while also being more explainable. However, tree-based models are difficult to accelerate with conventional digital hardware due to irregular memory access patterns. Here we show a tree-based ML accelerator based on a novel analog content addressable memory with memristor devices, capable of handling multiple types of bagging and boosting techniques common in tree-based algorithms. Our results show a large improvement of ∼60× lower latency and 160× reduced energy consumption compared to the state of the art, demonstrating the promise of our accelerator approach.