Details
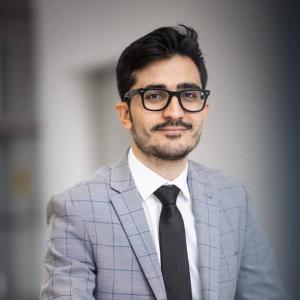
- Affiliation
-
AffiliationMemorial University of Newfoundland
- Country
The fogging effect, which always leads to pattern distortion in layout and in turn causes performance degradation, has been considered as a significant concern for wider adoption of electron beam lithography. In this work, we propose a reinforcement learning (RL) placement method that applies deep Q-learning to train a neural network as an agent. Different from the previous RL-based placement works, our proposed method uses a topological representation scheme that can advantageously render smaller search space in comparison to the currently popular absolute-coordinates-based representation. Our method focuses on the sensitive analog devices in mixed-signal ICs, which are better protected from potential variations due to fogging effects of other digital/analog portions.