Details
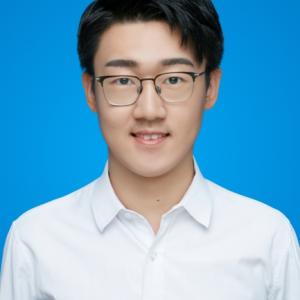
- Affiliation
-
AffiliationNanjing University
- Country
Distributed machine learning (ML) and other related techniques such as federated learning are facing a high risk of information leakage. Differential privacy (DP) is commonly used to protect privacy. However, it suffers from low accuracy due to the unbalanced data distribution in federated learning and additional noise brought by DP itself. In this paper, we propose a novel federated learning model that can protect data privacy from the gradient leakage attack and black-box membership inference attack (MIA). The proposed protection scheme makes the data hard to be reproduced and be distinguished from predictions. A small simulated attacker network is embedded as a regularization punishment to defend the malicious attacks. We further introduce a gradient modification method to secure the weight information and remedy the additional accuracy loss. The proposed privacy protection scheme was evaluated on MNIST and CIFAR-10, and compared with state-of-the-art DP-based federated learning models. Experimental results demonstrate that our model can successfully defend diverse external attacks to user-level privacy with negligible accuracy loss.