Details
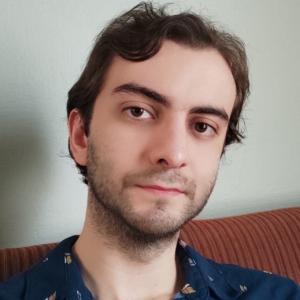
- Affiliation
-
AffiliationUniversidade Federal de Pelotas
- Country
The Affine Motion Estimation (AME) was introduced in the state-of-the-art Versatile Video Coding (VVC) standard to allow for the detection of non-translational transformations during inter-frame prediction. Although providing important coding efficiency gains, this new tool represents 43% of the motion estimation (ME) complexity. However, an analysis over the AME step shows that the Affine motion vectors are often generated without resulting in the best ME prediction. This paper proposes a AME early search termination based on supervised machine learning. Six Random Forest models were trained with features obtained during the encoding process to accurately predict whether the AME step should be executed, partially executed or skipped, avoiding unnecessary calculations. As result, the proposed solution achieves an average time saving of 46.94% in the AME step with a coding efficiency loss of only 0.18%.