Details
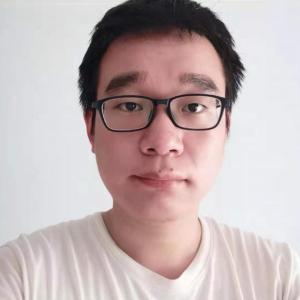
- Affiliation
-
AffiliationBeihang University
- Country
-
CountryChina
Adversarial attack has been a hot topic for a long time in machine learning and deep learning. Studying adversarial attack has vital significance to artificial intelligence security. Existing methods mainly pursue a higher attack success rate. Few researches pay attention to the region where adversarial perturbations are added. Actually, different pixels in an image usually have different contributions in results, which motivates us to apply region constraint in the image for adversarial perturbations generation. In this paper, we present an easy-to-implement way to decrease the unnecessary adversarial perturbations while preserving a relatively high attack success rate. Specifically, we do not use the same constraint of perturbations in the input image but set specific constraint for specific region. Furthermore, we point that adversarial examples work in a different way to normal images. Directly using the activated region in normal images is not optimal. Then, to get the crucial area in adversarial attacks, we propose six transformation schemes to revise the activated region which is generated by the normal image.