Details
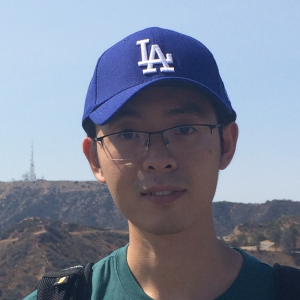
- Affiliation
-
AffiliationUniversity of California, Los Angeles
- Country
-
CountryUnited States
Recent study shows that the rat’s position on linear track can be decoded from the calcium images recorded from the hippocampal CA1 region of the rat’s brain. However, the real-time position decoding remains a challenge considering the complex decoding process and the strict requirements on hardware and energy cost for closed-loop feedback applications. In this paper, we propose two neural network based methods and corresponding hardware designs for realizing real-time position decoding from calcium images. Our methods are based on: 1) a simple convolutional neural network (CNN), and 2) a rate-based spiking neural network (SNN) converted from the CNN. We quantized the CNN and the SNN into 8-bit fixed point and implemented them on a low-power FPGA. Evaluation results show that the CNN and the SNN methods achieve 56.3%/83.1% and 56.0%/82.8% Hit-1/Hit-3 accuracy for the position decoding across different rats, respectively. We also observed an accuracy-latency tradeoff of the SNN method in decoding positions under various time steps. Finally, we present our SNN implementation on the neuromorphic chip Loihi.