Details
Presenter(s)
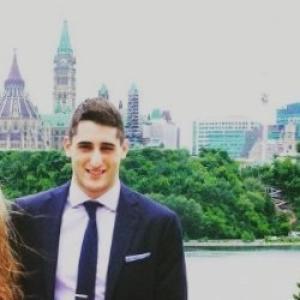
Display Name
Danilo Vucetic
- Affiliation
-
AffiliationMcGill University
- Country
-
CountryCanada
Abstract
We propose a method to reduce the fine-tuning costs of BERT-based natural language models such that they may be realized on resource-constrained devices. We identify memory usage as a major bottleneck and reduce memory operations during fine-tuning by training only a subset of the model. A reconfiguration of the model achieves better memory performance and training time. Our approach reduces memory usage, memory access time, and fine-tuning time substantially, while achieving near-baseline metric performance.