Details
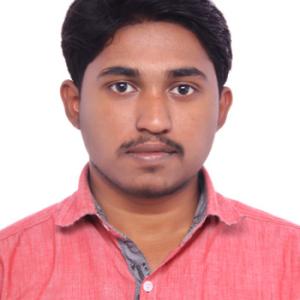
- Affiliation
-
AffiliationTCS Research
- Country
-
CountryIndia
In this paper, we propose EchoWrite-SNN, a robust edge compatible air-writing recognition system (used in applications such as AR/VR, HRI etc.) based on principles of SONAR and neuromorphic computing. The bare finger movements in air are captured by a pair of commonly available speaker-microphone pair. A new tracking algorithm based on windowed difference cross-correlation and ESPRIT is employed which shows better tracking accuracy compared to state-of-the-art methods with a median tracking error of only 3.31mm. To classify these air-written shapes, a 5-layer CNN is trained and then converted to a Spiking Neural Network (SNN) using ANN-to-SNN conversion technique to reap the benefits of low power neuromorphic computing on edge. Experimental results show that the converted SNN achieves 92% accuracy(a mere 3% less than the CNN) while showing 4.4x reduction in number of operations compared to CNN resulting in further energy benefit when run on actual neuromorphic computation platforms.