Details
Presenter(s)
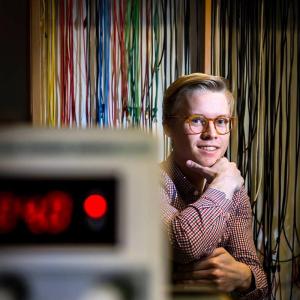
Display Name
Thorir Mar Ingolfsson
- Affiliation
-
AffiliationETH Zürich
- Country
-
CountrySwitzerland
Abstract
We propose a novel temporal convolutional network for cardiac arrhythmia detection that achieves 16.5% higher balanced accuracy than the SoA network, with 27x fewer parameters and 37x less operations. We implement our model on two platforms, the STM32L475 with ARM Cortex-M4F, and the GreenWavesTechnologies GAP8 with 1+8 RISC-V CV32E40P cores. Measurements show that GAP8 implementation respects real-time constraints while consuming 0.10mJ/inference. With 9.91 GMAC/s/W, it is 23x more energy-efficient and 46.85x faster than an ARM Cortex-M4F implementation. Overall, we obtain 8.1% higher accuracy while consuming 19.6x less energy and being 35.1x faster compared to a previous SoA embedded implementation.